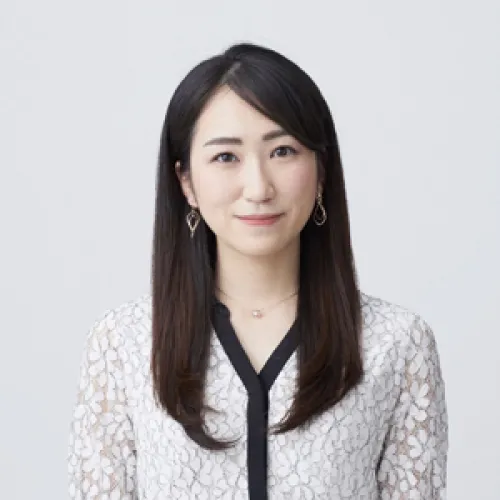
(she/her/hers)
University of Washington
NLP, Question Answering, Multilingual NLP, Reasoning
Akari Asai is a Ph.D. student in the Paul G. Allen School of Computer Science & Engineering at the University of Washington, advised by Prof. Hannaneh Hajishirzi. Her research lies in natural language processing and machine learning. Her recent research focuses on question answering, multilingual NLP, NLP efficiency. She received the IBM Fellowship in 2022 and the Nakajima Foundation Fellowship in 2019. Prior to UW, she obtained a B.E. degree in Electrical Engineering and Computer Science from the University of Tokyo.
Scalable Retrieval-Augmented Generation for Information Access for Everyone
The world's rapidly expanding web knowledge is being represented in diverse languages, modalities, and styles. My long-term research goal is to build models that interact with broad swaths of internet users to answer their questions, giving everyone equal access to the valuable information they need. Beneath the impressive progress in natural language processing (NLP), they are often exclusively built for English, require massive computational resources, lack complex reasoning abilities, and are brittle towards surface-level changes. I tackle these challenges to bridge the gap between NLP research and real-world applications. The theme of my research lies in three folds, multilingual NLP, efficient NLP, and neuro-symbolic NLP.
First, Information is not uniformly spread across different languages, therefore retrieving knowledge in a specific language might not be feasible. I introduce the first large-scale open-retrieval question answering dataset XOR-TyDi QA, covering 40k questions in 7 diverse languages. I further proposed the first unified multilingual retriever-generator framework, which effectively locates relevant Wikipedia passages beyond language boundaries and generates final answers conditioned on them with no translations, enabling to answer questions posed in 28 languages. Secondly, to overcome the const of computational and storage inefficiency, I introduce a method that massively reduces the storage requirements for neural retrieval models (60GB to 2GB) and a multi-task modular approach that efficiently transfers knowledge from multiple tasks stored in small task embeddings. I am currently working on a multi-task prompted retriever, which one can easily control a model's behaviors without any training. Lastly, to overcome the sensitivity of neural models, I incorporate symbolic approaches such as neural search over graph or first-order logic guided training regulation for complex questions and safety-critical applications.