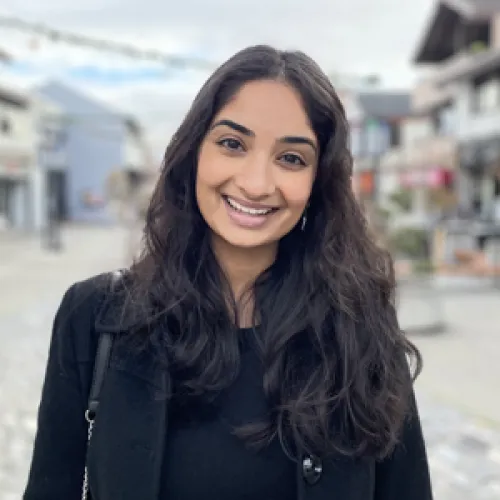
(she/her/hers)
University of California: Berkeley
Intelligent biomedical devices, hardware for machine learning, in-sensor biosignal recognition, brain-inspired computing
Alisha's research is in the area of neural engineering, an interdisciplinary field centered on the interface between humans and computers. Her focus is on digital integrated circuits and systems for biomedical applications, specifically the intersection of hardware-efficient machine learning algorithms, biosignal sensor fusion and classification, gesture recognition, and closed-loop neural prosthetic feedback. During her Ph.D. she has published in several conferences and journals regarding highly efficient machine learning for biosignals through brain inspired computing, and on its applications in robotic navigation and assistive prosthetics. Alisha won the NSF Graduate Research Fellowship and UC Berkeley Fellowship in 2018, and she received the prestigious UC Berkeley Outstanding Graduate Peer Mentor award in 2022 for outstanding commitment to mentoring & advising undergraduate students, and fostering inclusivity; she is the first awardee from the college of engineering.
Augmented Prosthetics through Highly-efficient Brain-inspired Learning & Shared Control
Brain-inspired hyperdimensional computing (HDC) has shown promise for highly accurate biosignal classification and robotic navigation owing to its few-shot learning capabilities, and robustness to noise and electrode placement variability. The simplistic paradigm is also ultra low-power and low latency, as shown in our prior work, potentially enabling local and fast closed-loop on-device control of assistive prosthetic devices. This work proposes to achieve this through a user-adaptable, multi-layer shared control scheme that executes on the user's goal while alleviating the burden of fine control. The lower layers were designed to efficiently recognize and predict the user's prior and future intents with greater than 90% accuracy based on EMG and accelerometer sensor data, while shared control is achieved in the highest level that maps user-intent and prosthetic finger force sensor data to robotic actuation.