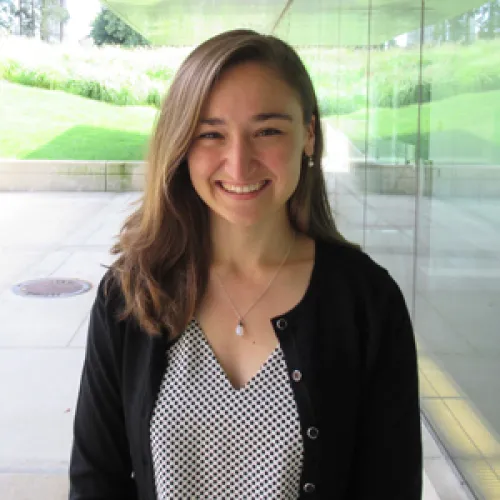
(she/her/hers)
Argonne National Laboratory
power and energy systems, optimization, control, machine learning, infrastructure resilience
Alyssa Kody is a Maria Goeppert Mayer Postdoctoral Fellow at Argonne National Laboratory in Lemont, Illinois. Her research focuses on developing control and optimization algorithms for power and energy systems. She received her Ph.D. in Electrical Engineering from the University of Michigan in Ann Arbor in 2019, where her thesis was on developing control systems for self-powered technologies. Her graduate work was supported by a National Science Foundation Graduate Research Fellowship and a Rackham Merit Fellowship.
Machine-learning-enhanced optimization for power systems
The electric power grid of the future faces many challenges (e.g., incorporating high penetrations of distributed energy resources, and a need for greater resilience to natural disasters). Conventional grid optimization and control techniques, which often rely on assumptions and simplifications of physical system models for computational tractability, may not be able to meet the accuracy necessary to provide efficient, reliable, and cost-effective power. Machine learning (ML) offers promising data-driven techniques that may provide a means of increasing computational tractability and accuracy of power systems algorithms. However, most ML methods act as black-boxes, and for power systems applications, this can lead to security or robustness issues with large societal consequences. In my research, I develop ML-enhanced optimization algorithms for power systems, where I target the use of ML to improve computation speed or accuracy of operation algorithms. I will present several examples of these works. First, in power systems applications, the performance of many distributed optimization algorithms strongly depends on heuristically-chosen parameters. We used reinforcement learning (RL) to develop a parameter selection policy for the nonlinear AC optimal power flow problem that significantly accelerates convergence and shows promise for generalizability to unseen operating conditions. Second, the AC unit commitment problem is a notoriously challenging mixed-integer nonlinear program (MINLP). We trained a neural network to model the nonlinear AC power flow equations to obtain a tractable mixed-integer linear program (MILP) and embed this model in AC unit commitment problems. This method transforms the AC unit commitment problem into a more manageable MILP that outperforms previous approximations. Finally, I will present how targeted ML can be applied to challenging wildfire risk mitigation problems for power systems.