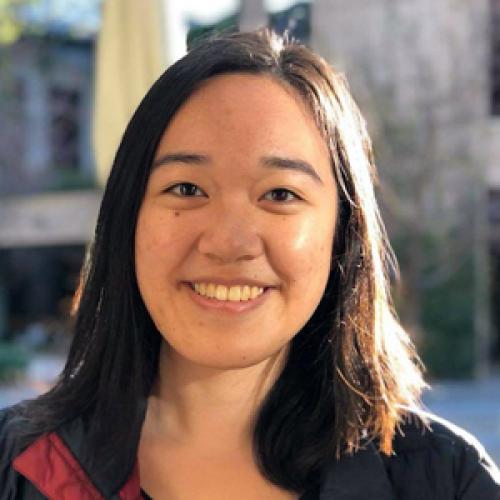
(she/her/hers)
Stanford University
robotics, artificial intelligence, reinforcement learning
Annie Xie is a PhD student in Computer Science at Stanford University advised by Chelsea Finn. Before Stanford, she received her bachelor's degree with High Honors in Electrical Engineering and Computer Science from UC Berkeley. Her research focuses on developing robotic systems that learn with minimal human supervision. She is a recipient of the NSF Graduate Research Fellowship.
Robotic Reinforcement Learning under Continual Non-Stationarity
As humans, our goals and our environment are persistently changing throughout our lifetime based on our experiences, actions, and internal and external drives. In contrast, typical robotic learning problem set-ups consider decision processes that are stationary across episodes. Can we develop robotic reinforcement learning algorithms that can cope with the persistent change in the former, more realistic problem settings? In this work, we formalize this problem setting, and draw upon ideas from the online learning and probabilistic inference literature to derive an off-policy RL algorithm that can reason about and tackle such lifelong non-stationarity. Our method leverages latent variable models to learn a representation of the environment from current and past experiences, and performs off-policy RL with this representation. We empirically evaluate our method on several environments that exhibit continual non-stationarity. Finally, we demonstrate our method in multi-agent and human-robot settings, where non-stationarity arises as a result of other agents.