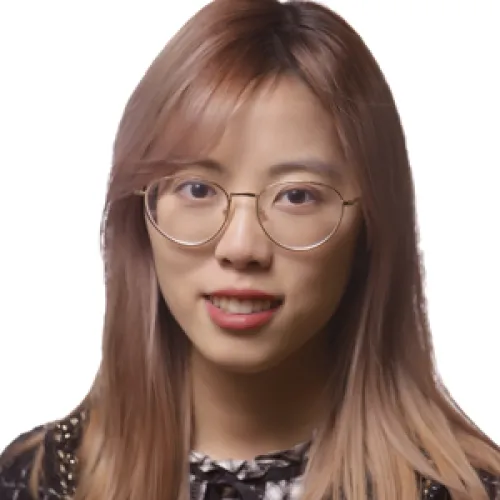
(she/her/hers)
University of Michigan
Human Computer Interaction, Programming and Development Support, Educational Technology, Human-AI Interaction, Data Science
April Wang is a Ph.D. candidate at the School of Information at the University of Michigan, advised by professor Steve Oney and professor Christopher Brooks. With the growing complexity and interdisciplinary of the data science field, data science workers must embrace effective collaboration to improve the quality and efficiency of the work. Her work aims to understand different collaboration needs and challenges around data science, and design better programming tools to support collaborative data science. Her work has been published at top-tier HCI venues (e.g., CHI, TOCHI, CSCW), and has received several paper awards. She received B.Eng. in Computer Science from Zhejiang University and M.Sc. degree in Computer Science from Simon Fraser University.
Designing Future Computational Notebooks for Collaboration and Learning
Computing technologies allow people to work, learn, and socialize remotely but seamlessly together, particularly over complex computational tasks like programming and data science. Yet, collaboration in data science is often hard. Since data science is highly exploratory, the artifact and analysis often iterate fast. It is difficult to maintain a shared understanding across various collaborators. On the other hand, tools like computational notebooks provide a convenient approach for data scientists to run, document, and share analysis in a storytelling way. However, there are still many open-ended questions about how to improve the collaboration experience by designing better collaborative data science tools. For example, data scientists often neglect to keep updated documentation during rapid exploration, which results in computational notebooks that are messy and difficult to read; without strategic planning, working together in a shared notebook may block each other's work. My research draws upon human-centered design techniques to identify barriers in real-world data science programming practices, and explore the design space of collaborative data science environments through tool-building.