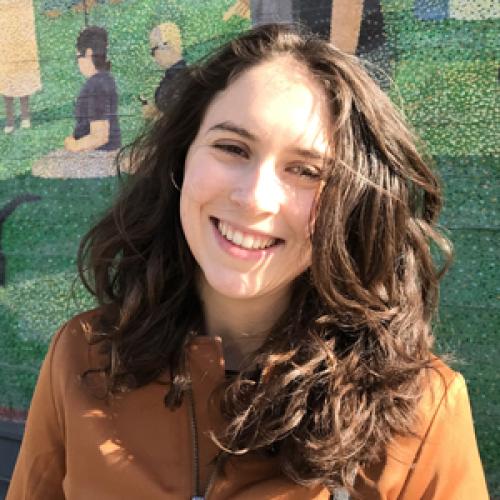
(she/her/hers)
California Institute of Technology
Optimal and Robust Control, Distributed Control, Data-driven Control, Convex Optimization, Large-scale Systems.
Carmen Amo Alonso s currently a Ph.D. candidate in Control and Dynamical Systems at Caltech, working with Prof. John Doyle. During her PhD, Carmen has been honored to receive an AI4Science Amazon fellowship and a D.E. Shaw Exploration fellowship. Prior to starting her PhD, she received a B.Sc. in Aerospace Engineering from the Polytechnic University of Madrid (Spain) in 2016, and a M.Sc. in Space Engineering from Caltech in 2017. Her research interests include Distributed Optimal Control, Convex Optimization, and Large-Scale Systems. More recently, she has been working on applying ideas of control theory to computational linguistics.
Distributed and Localized Model Predictive Control
The increasing presence of large-scale distributed cyber-physical systems highlights the need for scalable control strategies where only local communication is required. Moreover, in safety-critical systems it is imperative that such control strategies handle constraints in the presence of disturbances. In response to this need, the Distributed and Localized Model Predictive Control (DLMPC) framework is a suitable solution for large-scale linear systems. DLMPC is a distributed closed-loop model predictive control scheme wherein only local state and model information needs to be exchanged between subsystems for the computation and implementation of control actions. We use the System Level Synthesis framework to reformulate the centralized MPC problem, and show that this allows us to naturally impose localized communication constraints between sub-controllers. The structure of the resulting problem can be exploited to develop a distributed and localized computation of closed-loop control policies. In this framework, computational complexity of the subproblems solved by each subsystem in DLMPC is independent of the size of the global system. Moreover, this approach is robust to disturbances, enjoys recursive feasibility and asymptotic stability guarantees, and has extensions to the data-driven setting.