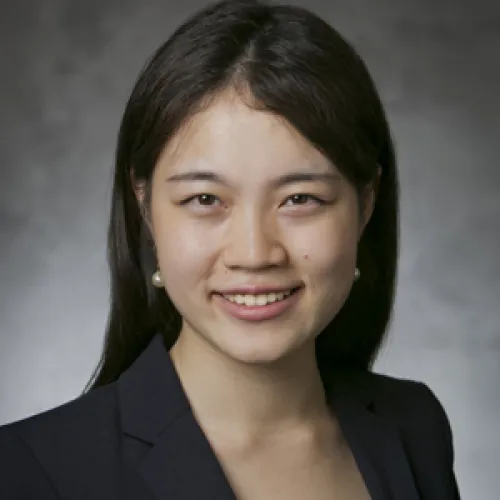
(she/her/hers)
Carnegie Mellon University
Computational biology, single-cell genomics, spatial-temporal analysis
Dongshunyi "Dora" Li is a Ph.D. Candidate in the Computational Biology Department of the School of Computer Science at Carnegie Mellon University. Her research focuses on developing robust, scalable and interpretable machine learning methods to uncover biological mechanisms using high-throughput sequencing data. She is currently working with Prof. Ziv Bar-Joseph on developing methods to study cell-cell interactions and their impact on dynamic biological processes using spatio-temporal single cell data. Prior to Carnegie Mellon, she received her master's degree in biostatistics from Duke University, where she worked with Prof. Raluca Gordan on developing statistical methods modeling the impact from DNA mutations on transcription factors binding. She received her B.S. in Biochemistry from the Hong Kong University of Science and Technology.
Spatio-Temporal Analysis of Single Cell Data
One of the fundamental questions in biology is how cells interact and differentiate to generate tissues, organs and ultimately the entire human body. Many studies have contributed to our understanding of this developmental process. However, it is only until recently that we can study this problem in a systematic and data-driven manner. Thanks to
the sequencing technologies, we can now access tens of thousands of features characterizing numerous numbers of cells in their original locations within tissues over time. These large amount of high-dimensional spatio-temporal data provide unprecedented opportunities to study cell-cell interactions and their impact on the developmental process. On the other hand, they also pose unique computational and analytical challenges. My research centers on developing scalable, robust and interpretable machine learning models using spatio-temporal single cell data to identify cells and to unveil their interaction mechanisms and the roles of these in the developmental process. We first proposed an unsupervised method based on autoencoders to simultaneously cluster and annotate cells using prior knowledge of biological processes. This makes the method robust to noise and outperform others. The identified biological processes for cells provide strong evidence for their corresponding cell types. Given inferred cell types, we then proposed descriptive statistics and significance tests based on temporal covariance to study cell type - cell type interactions. We experimentally tested our results and showed that our method identified interactions crucial to developmental processes. To move from interactions between cell types to individual cells, we next incorporated cells' original locations in tissues. We developed a multi-task Mixture-of-Experts model to learn interaction signals among nearby cells and how these impact cell states. We showed the model achieves high prediction accuracy while being fully interpretable. The signals identified play important roles in cell-cell interactions and can shed light on the impact of these interactions on cell states.