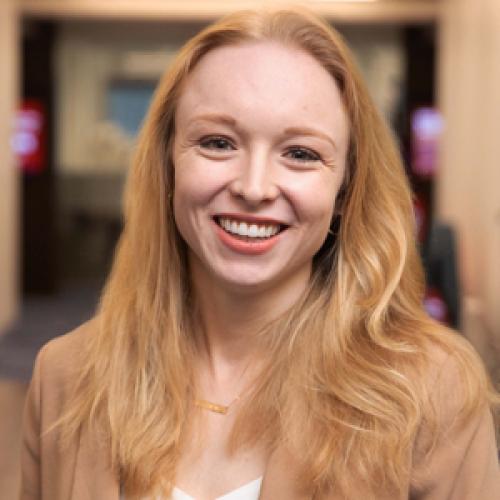
(she/her/hers)
University of Chicago
machine learning, security, privacy
Emily Wenger is a final year computer science PhD student at the University of Chicago, advised by Ben Zhao and Heather Zheng. Her research focuses on security and privacy issues of machine learning systems. Her work has been published at top computer security (CCS, USENIX, Oakland) and machine learning (NeurIPS, CVPR) conferences and has been covered by media outlets including the New York Times, MIT Tech Review, and Nature. She is the recipient of the GFSD, Harvey, and University of Chicago Neubauer fellowships. Previously, she worked for the US Department of Defense and interned at Meta AI Research. 
Reclaiming Data Agency in the Age of Ubiquitous Machine Learning
As machine learning (ML) models have expanded in size and scope in recent years, so has the amount of data needed to train them. This creates privacy risks for individuals whose data -- be it their images, emails, tweets, or browsing history -- is used for training. For example, ML models can memorize their training data, revealing private information about individuals in the dataset. Furthermore, users whose data is co-opted for ML use may end up enrolled in a privacy-compromising system, such as a large-scale facial recognition model. Most existing work on ML data privacy accepts the premise that data use is inevitable and instead tries to mitigate privacy risks during model training. However, privacy-conscious individuals may desire agency over how and if their data is used, rather than only having their privacy preserved when it is used. Data agency, the ability to know and control how and if one's data is used in ML systems, is an important complement to existing privacy protection approaches, and it is the focus of my research.
Data agency can take many forms, and my research in this area focuses on developing technical solutions that enable individuals to disrupt or discover when their data is used in large-scale ML systems. My current research targets data agency in the context of large-scale facial recognition (FR) systems, providing ways for users to combat unwanted facial recognition. A secondary line of research assesses real world security and privacy threats posed by large-scale ML systems.