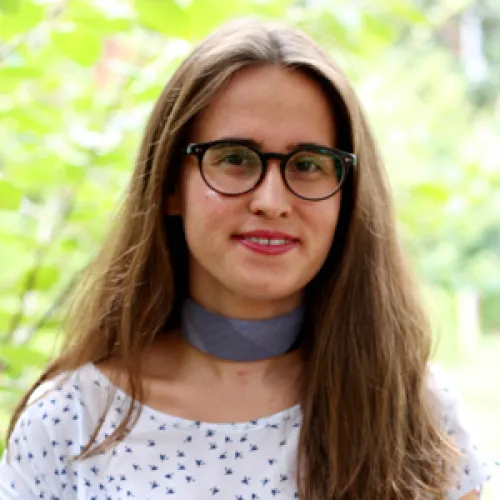
(she/her/hers)
Stanford University
Robotics. Assured autonomy. Safety monitoring and validation. Planning under uncertainty
Esen Yel is a Postdoctoral Scholar at Stanford University, advised by Mykel Kochenderfer. She obtained her Ph.D. in System Engineering from the University of Virginia in 2021. Her research focuses on planning, safety monitoring and validation, and online learning for autonomous systems under uncertainties. Specifically, she aims to build autonomous systems that can make accurate predictions under uncertainties and make robust and safe decisions accordingly. Her research has been presented at Robotics Science and Systems Pioneers workshop and she is the recipient of the Link Lab Outstanding Graduate Research award and the Ruthie Oxford Memorial Award. Her work has been published in various robotics venues including the Robotics and Automation Magazine (RAM) and Robotics and Automation Letters (RA-L). Esen earned her M.S. and B.S. degrees in Electrical Engineering from Bogazici University in Turkey.
Predictive Planning and Runtime Monitoring for Safe Autonomous Operations under Uncertainties
Autonomous systems are being used in many applications, including transportation, inspection, and healthcare, thanks to their promise of an increase in efficiency and performance. In critical settings, these systems need to operate safely and reliably to prevent disastrous consequences. However, real-world conditions bring many challenges threatening safety, including uncertainties (e.g., environmental disturbances, sensing noises, etc.). Real-world is also very dynamic, requiring systems to reliably adapt to changes in the environment settings. My main research goal is to build autonomous systems that make accurate predictions under uncertainties and make robust and safe decisions accordingly. My research combines tools from reachability analysis, machine learning and verification to provide runtime safety monitoring for autonomous systems under uncertainties. Moreover, I develop planning techniques that use online learning and transfer learning to adapt to new tasks or environments. By applying these techniques in real vehicles or realistic simulators, I demonstrate safety improvements under various uncertainties, including external disturbances, sensing uncertainties, and unforeseen faults and tasks.