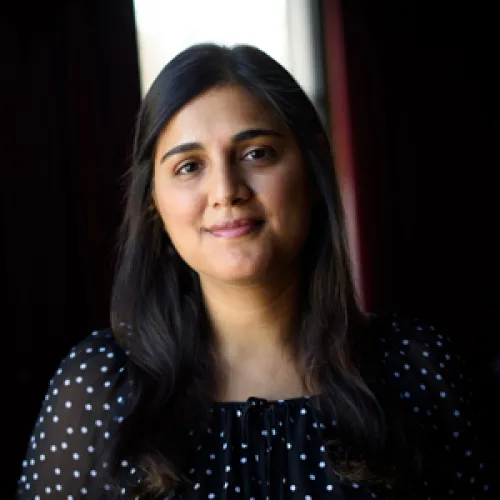
(she/her/hers)
University of Michigan
Human-AI Collaboration, Interpretable ML, Sensemaking, Cognitive Science
Harman Kaur is a PhD candidate in both the department of Computer Science and the School of Information at the University of Michigan, where she is advised by Eric Gilbert and Cliff Lampe. Her research interests lie in human-AI collaboration and interpretable ML. Specifically, she studies interpretability tools from a human-centered perspective and designs solutions to support the bounded nature of human rationality in the context of ML-based decision-support systems. She has published several papers at top-tier human-computer interaction venues, such as CHI, CSCW, IUI, and FAccT. She has also completed several internships at Microsoft Research and the Allen Institute for AI. Prior to Michigan, Harman received a BS in Computer Science from the University of Minnesota.
Leveraging Human Cognition in AI Interaction
When designing for effective human-AI interaction, we cannot assume that people are entirely rational agents. Yet, much of the scholarship to this point has focused on developing AI that can explain itself (e.g., via interpretability tools), under the assumption that people perfectly internalize this information and, as a result, are able to better interact with their AI counterpart. In my work, I argue that helping people understand AI is as much a human problem as a technical one: it is bound by both people's information processing capabilities and the quality of information AI can provide. I study human-AI interaction in the context of ML-based decision-support systems. My work has focused on identifying the challenges in interpretability tool use, observing cognitive and social heuristics as a barrier to people's understanding of ML models. These heuristics serve as automatic shortcuts that make people's decision-making tasks easier and faster to accomplish, without having to reason about the information being presented. Looking ahead, my goal is to incorporate theories from cognitive science and organizational science--fields that study individual and collective human sensemaking--for designing solutions that better support people's understanding of AI.