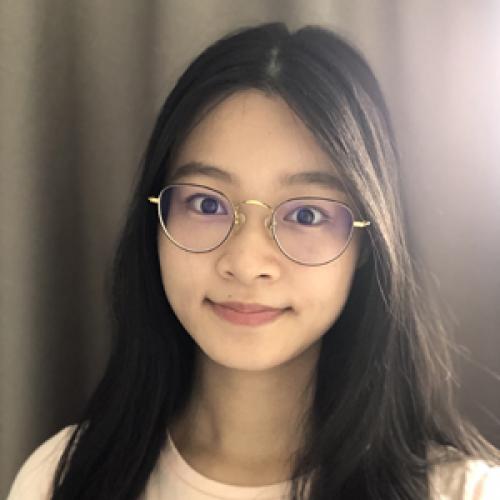
(she/her/hers)
National University of Singapore
Information theory, statistical learning theory, inference and estimation
Haiyun He is currently a Ph.D. candidate in the Department of Electrical and Computer Engineering (ECE) at the National University of Singapore (NUS). She has successfully defended her thesis in August and will receive the degree at end September. From Sep 2017 to Jul 2018, she was first a Research Assistant in ECE at NUS. She received the B.E. degree in Beihang University (BUAA) in 2016 and the M.Sc. (Electrical Engineering) degree in ECE from NUS in 2017. Her research interests include information theory, statistical learning and their applications.
FUNDAMENTAL PERFORMANCE LIMITS OF STATISTICAL PROBLEMS: FROM DETECTION THEORY TO SEMI-SUPERVISED LEARNING
Studying and designing close-to-optimal mechanisms to infer or learn useful information from raw data is of tremendous significance in this digital era. Our work explores the fundamental performance limits of three classes of statistical problems--distributed detection, change-point detection and the generalization capabilities of semi-supervised learning (SSL). In a sensor network, the distributed detection problem concerns the scenario in which the fusion center needs to make a decision based on data sent from a number of sensors via different channels. The change-point detection problem concerns the scenario in which the data samples are collected under different conditions and one needs to estimate the change-points of the condition. In contrast to classical works where the underlying data distributions are assumed to be known, we consider the practical scenario where training data samples are available instead. For distributed detection, we derive the asymptotically optimal type-II error exponent given that the type-I error decays exponentially fast. We also derive the asymptotically optimal test at the fusion center. For change-point detection, we derive the asymptotically optimal change-point estimator in both large and moderate deviations regimes, as well as the asymptotically optimal detection confidence width as a function of the undetected error. Finally, we consider a more complicated scenario in which we are motivated to mitigate the high cost of labelling data. To do so, we analyse the fundamental limits of SSL which makes use of both labelled and unlabelled data. Using information-theoretic principles, we investigate the generalization performance of SSL, which quantifies the extent to which the algorithms overfits to the training data. We show that under iterative SSL with pseudo-labelling, for easier-to-distinguish classes, the generalization error decreases rapidly in the first few iterations and saturates afterwards while for difficult-to- distinguish classes, the generalization error increases instead. Regularization can help to mitigate this undesirable effect. Our experiments on benchmark datasets such as the MNIST and CIFAR-10 datasets corroborate our theoretical results.