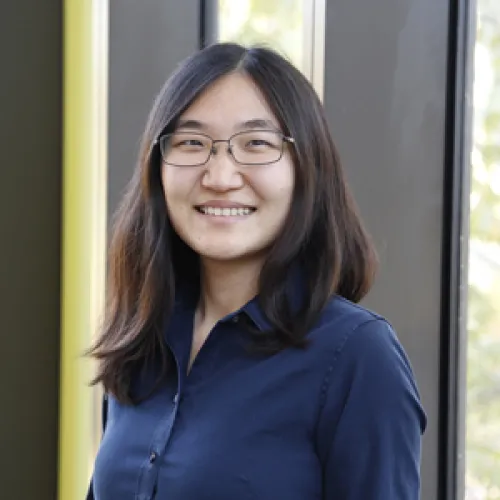
(she/her/hers)
Caltech
AI for science, Human-in-the-loop systems, Machine learning, Computer vision
Jennifer is a PhD candidate in Computing and Mathematical Sciences at Caltech, advised by Professors Pietro Perona and Yisong Yue. Her research focuses on developing computational systems for scientists, especially in workflows that require expert-in-the-loop interactions, such as for studying behavioral, affective, or medical data. She aims to accelerate AI-assisted scientific discovery and optimize expert attention in real-world workflows, addressing bottlenecks including data annotation efficiency, model interpretability and generalization, and semantic structure discovery. Beyond her research work, she has organized multiple workshops to facilitate connections across fields at top AI conferences, such as CVPR, and she has received multiple awards, such as best student paper at CVPR 2021.
AI for Scientists: Learning from Experts and Data
Scientific discoveries, built upon experiments, have created solutions to global problems and fundamentally improved our world. With rapidly increasing amounts of experimental data, machine learning becomes more and more crucial for automating scientific data analysis. However, many real-world workflows demand expert-in-the-loop attention and require models that not only interface with data, but also with experts such as behavioral neuroscientists and medical doctors. My research centers around expert-in-the-loop frameworks that bridge machine learning and real-world use cases, to enable scalable interpretation of scientific insight from data. These models must accommodate vastly varying modalities, analysis tasks, and existing knowledge inherent in scientific domains, while optimizing expert effort in the workflow. My approach is to understand the interface between data, models, and scientists across domains, and apply this understanding to develop more efficient methods, such as for discovering semantically meaningful structure from data and integrating domain knowledge with learning. I collaborate closely with scientists to integrate these methods in practice to accelerate scientific progress.