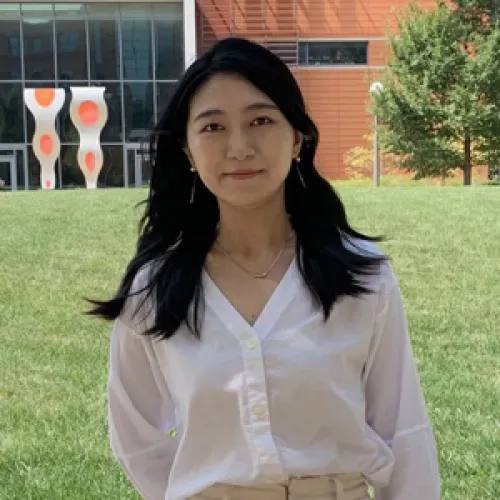
(she/her/hers)
University of Illinois Urbana-Champaign
Natural Language Processing, Multimedia Knowledge, Information Extraction
Manling Li is a fifth-year Ph.D. student at the Computer Science Department of University of Illinois Urbana-Champaign. Her work on multimedia knowledge extraction won the ACL'20 Best Demo Paper Award and NAACL'21 Best Demo Paper Award. She was selected as a DARPA Riser in 2022. She was a recipient of Microsoft Research PhD Fellowship. She was awarded C.L. Dave and Jane W.S. Liu Award, and has been selected as Mavis Future Faculty Fellow. She has more than 30 publications on multimedia knowledge extraction and reasoning, and gave tutorials about multimedia information extraction at AAAI'21, ACL'21 and NAACL'22. Additional information is available at https://limanling.github.io.
Multimedia Event Knowledge Acquisition: From Object-Centric to Event-Centric Understanding
Human memories can be viewed as repositories of historical events. An event is a semantic structure encapsulating the fundamental questions of Who? What? Where? When? Why?, which are of primary concern to humans. The enormous volume of data requires machines to automatically obtain such factual knowledge including events and their arguments (participants) and perform reasoning by synthesizing a wide variety of unstructured data, such as text, images, and videos. However, current event understanding is text-only, local, and lacking in reasoning.
This talk focuses on constructing event graphs to deal with real-world events that are multimedia, interconnected, probabilistic, and span a long time period. We discover the local event structures (i.e., who, what, where, and when) by transferring the semantic understanding ability from text to vision during vision-language pretraining. To perform global reasoning across events (i.e., what is likely to occur, and why), we further investigate the interactions between events such as the temporal orders and the evolution patterns along a long period, and leverage historical events to discover such event schema knowledge globally. Our structural event graph modeling is able to represent the global inter-dependencies of events and long-distance interactions via arguments, leading to a comprehensive understanding of events and effective forecasting of future events.