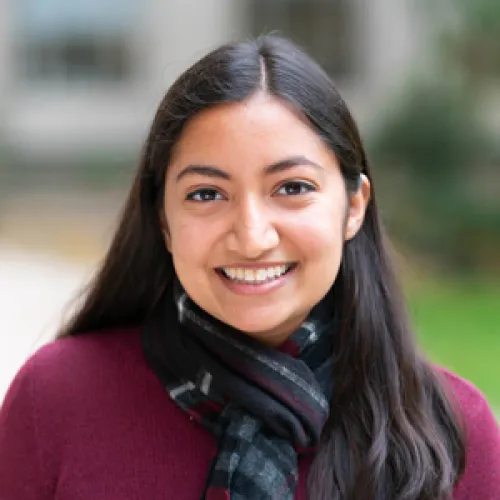
(she/her/hers)
Massachusetts Institute of Technology
machine learning, healthcare, natural language processing
Monica Agrawal is a PhD candidate in Computer Science at MIT CSAIL, advised by Professor David Sontag in the Clinical Machine Learning Group. In her research, she tackles diverse challenges across clinical natural language processing. Her work has been published in machine learning conferences (AISTATS, ICML), human-computer interaction conferences (CHI, UIST), and clinical/biomedical venues (JCO Clinical Cancer Informatics, Bioinformatics, Machine Learning for Healthcare). She has been the recipient of a Takeda Fellowship, a Tau Beta Pi Fellowship, and an MIT EECS Edgerton Fellowship. Previously, she graduated from Stanford with a BS and MS in Computer Science and held internships at Google and Flatiron Health.
Generating high-quality structured data from clinical notes
The adoption of electronic health records (EHRs) presents an incredible opportunity to move us towards the future of medicine – by catalyzing retrospective clinical research and enabling personalized clinical decision support. However, this promise is far from fully realized, in part because much of our health data is trapped within unstructured free text clinical notes. In my research, I develop novel machine learning and natural language processing algorithms to generate high-quality structured medical data from clinical text. At the heart of this research are close collaborations with clinicians and experts in human-computer interaction.
Given patient privacy concerns and the jargon-heavy dialect underlying clinical text, there is often only little labeled data available. My research develops few-shot extraction methods requiring minimal supervision. This has involved designing a new self-supervision objective tailored for longitudinal text data and leveraging advances in large language models. Given their high-stakes nature, certain clinical applications can require near perfect performance. Given the fallibility of algorithms and the complexity of healthcare, my research has additionally developed a hybrid human-ML framework to de-risk the use of machine learning and quantitatively studied the effect of decision aid on clinicians. While the bulk of my research focuses on transforming existing clinical data into a more structured format, the data would ideally be made structured at the time of creation. The last prong of my research involves augmenting EHR interfaces with machine learning tooling (e.g. autocomplete, contextual information retrieval) to incentivize the insertion of structured concepts. The goal of this work is to redesign clinical documentation to generate cleaner data while simultaneously saving clinicians' time. Overall, my research aims to harness the potential of clinical text to enable large clinical studies and decrease clinician burden