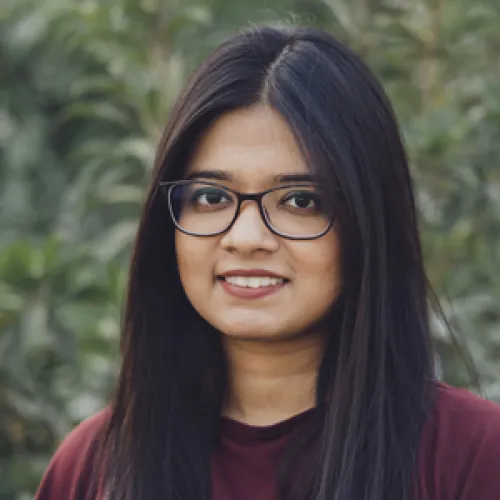
(she/her/hers)
Ph.D. Candidate, University of Missouri
low-power circuit design, VLSI, ML hardware accelerators , biomedical instrumentation and neuromorphic computing
Omiya Hassan is currently working as a graduate instructor and pursuing her Ph.D. in Electrical Engineering supervised by Prof. Syed Kamrul Islam, who is the chair of the in the department of Electrical Engineering and Computer Science (EECS) at the University of Missouri. She received her Bachelor of Science degree in Electrical Engineering in 2017 from United International University, Bangladesh and served as a lecturer at Presidency University, Bangladesh in 2018. Her current research topic focuses on designing power efficient machine-learning (ML) model based integrated hardware and circuits for biomedical applications and her research interest includes low-power circuit design, VLSI, biomedical system design, neuromorphic computing, and ML hardware accelerators. Ms. Hassan has received the IEEE Instrumentation and Measurement Society's Graduate Research Fellowship Award in 2021, the 1907 Women in Engineering Student Award in 2019, the Outstanding Doctoral Student Award from the Department of EECS at the University of Missouri in 2021 and the Research in Excellence award from the Graduate Professional Council at the University of Missouri in 2022. Throughout her Ph. D she has published multiple papers as author and co-author of leading conferences organized by IEEE (Institute of Electrical and Electronics engineering), journal paper in multiple peer-reviewed journal and even co-authored a book chapter with her colleagues and collaborators. She also worked as a Research Scientist Intern at Meta Reality labs in 2022. She has mentored and co-supervised a number of undergraduate students which led her to win the annual Outstanding Undergraduate Research Mentor of the year 2022 by the University of Missouri. She is in preparation to complete her Ph.D. journey by May 2023 and create her career in academia.
Power Efficient Neural Network Based Hardware Architectures for Real-Time Sleep Apnea Detection
The future of critical health diagnosis will involve intelligent and smart devices that are low-cost, wearable, and lightweight requiring power efficient hardware platforms. Various machine learning models such as deep learning architectures have been employed in the design of intelligent systems. However, the deployment of these sophisticated and intelligent devices in real-time embedded systems with limited hardware resources and power budget is difficult due to the requirement of high computational power in achieving high accuracy rate. As a result, this creates a significant gap between the advancement of computing technology and the associated device technologies for healthcare applications. To address this issue, this research introduces power efficient machine learning based digital hardware design techniques for a compact design solution while maintaining its optimal prediction accuracy. A hardware design approach called SABiNN is proposed and analyzed in this work which is a 2's complement based binarized digital hardware technique. Neural network models such as feedforward models are selected to analyze these proposed methodologies. Deep compression learning techniques for hardware implementation such as n-bit integer quantization, and deterministic binarization only on hyper-parameters are also employed. Instead of using matrix multiplication based multiply accumulate (MAC) function typically used in hardware accelerators, shifters and 2's complements are introduced which significantly reduces the power consumption rate by 5x. Moreover, during inference there is no need of internal nor external memory devices for storing the networks' weights and biases due to fixed hyper-parametric values. As a result, the processing power and model size are significantly reduced. For efficient use of these techniques in biomedical applications a sleep apnea detection device is developed which can detect sleep apnea (SA) in real-time. The input to the system consists of two physiological sensor data such as ECG signal from the chest movement and SpO2 measurement from pulse oximeter to predict the occurrence of SA episodes. In training phase, real patient data was used, and the network model was converted into the proposed hardware model to achieve targeted accuracy over 75%. During inference, all the parameters are successfully extracted, and each component was designed into re-programmable digital logic hardware before translated onto CMOS platform using 130nm and 180nm design processes.