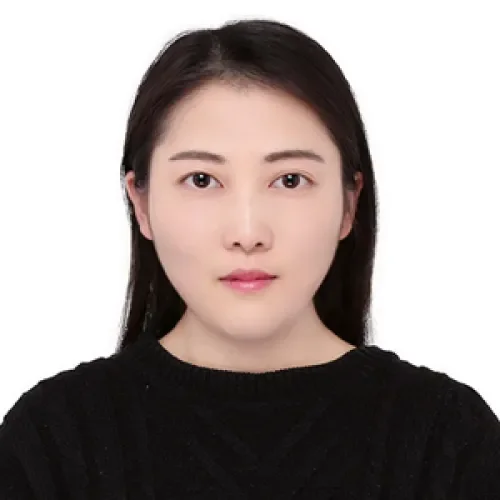
(she/her/hers)
George Mason University
Decentralized online kernel learning, random feature mapping, linearized ADMM, communication-censoring, quantization
Ping Xu is a postdoctoral research associate at the ECE department of George Mason University (GMU) advised by Professor Zhi (Gerry) Tian, where she received her Ph.D. in May 2022 and a M.S. in 2018. Prior to GMU, Ping obtained a B.S. from Northwestern Polytechnical University, Xi'an, Shaanxi, China. Her research interests span the areas of cooperative control, network optimization, data science, and machine learning, along with their applications in social, environmental, and IoT systems. Specifically, Ping is skilled in the analysis and control of complex distributed and/or networked systems and learning from large volume of networked data to make advances in science and engineering.
Communication-efficient Online Decentralized Kernel Learning
This work focuses on online kernel learning over a decentralized network. Each agent in the network receives continuous streaming data locally and works collaboratively to learn a nonlinear prediction function that is globally optimal in the reproducing kernel Hilbert space with respect to the total instantaneous costs of all agents. In order to circumvent the curse of dimensionality issue in traditional online kernel learning, we utilize random feature (RF) mapping to convert the non-parametric kernel learning problem into a fixed-length parametric one in the RF space. We then propose a novel learning framework named Online Decentralized Kernel learning via Linearized ADMM (ODKLA) to efficiently solve the online decentralized kernel learning problem. To further improve the communication efficiency, we add the quantization and censoring strategies in the communication stage and develop the Quantized and Communication-censored ODKLA (QC-ODKLA) algorithm. We theoretically prove that both ODKLA and QC-ODKLA can achieve the optimal sublinear regret over time slots. Through numerical experiments, we evaluate the learning effectiveness, communication, and computation efficiencies of the proposed methods.