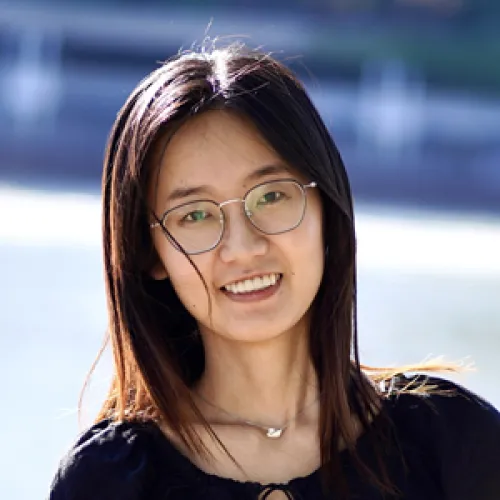
(she/her/hers)
Cornell University
Computer Vision
Qianqian Wang is PhD candidate at the Department of Computer Science at Cornell University, advised by Prof. Noah Snavely and Bharath Hariharan. Her research lies at the intersection of 3D computer vision, computer graphics, and machine learning. She is interested in understanding and modeling the physical proprieties of the 3D world using visual data, with a focus on reconstructing the 3D geometry of a scene and rendering high quality images for the scene. She is a recipient of Google PhD fellowship in 2022. She received B.S. degree in Information Engineering from Zhejiang University.
Reconstructing and Rendering the 3D World from Visual Data
Reconstructing and rendering the 3D world around us with compelling visual realism is an important problem in computer vision and graphics, with many applications in AR, VR, and content creation. Typically, this would either require a large amount of manual work from 3D artists, or scanning the scene carefully with expensive sensors, which restricts the applications to certain scenarios like gaming or filming. My research goal is to develop algorithms that can scale up to handle large-scale scenes and allow average users to capture and create their own 3D contents. To do so, I believe we will need to leverage image-based modeling and rendering (IBMR), which takes images or videos as input and produces 3D models and novel views of the scene. IBMR can model the world from just visual data, avoiding the need for manual modeling, leading to better scalability and accessibility.
My research focuses on improving IBMR by leveraging the increasingly available public visual data and the significant progress in machine learning and differentiable rendering techniques. Specifically, I developed a weakly supervised approach to learn correspondences from large amounts of data for 3D reconstruction, which enables accurate estimation of camera poses and scene geometry. I also devised a new image-based rendering approach that is able to generalize to unseen scenes while achieving high rendering quality. While we have great solutions for reconstructing and rendering static scenes, handling dynamic scenes is far more under-constrained and challenging. My ongoing work tries to understand long-range motion in videos, which is my first step towards understanding and modeling dynamic scenes from visual data.