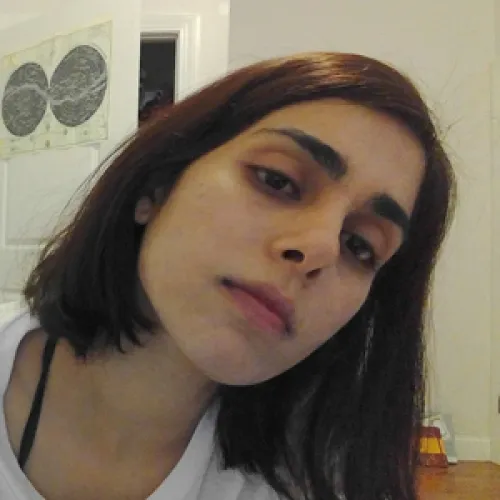
(she/her/hers)
Brown University
natural language, multi-agent communication, reinforcement learning
Roma Patel is a PhD student in Computer Science at Brown University, where her research focuses on grounded language learning i.e., teaching agents to understand and use language for more intelligent behaviour. More broadly, her research focuses on building agents that exhibit human-level intelligence to cooperatively solve tasks by using insights from the study of language. Given the interdisciplinary nature of this research agenda, Roma's research spans the fields of robotics, multi-agent reinforcement learning and natural language processing. Her work has been published at top-tier conferences such as ICLR, ICML, RSS, ACL, EMNLP etc. Apart from this, she has also participated in organising and program committees for several workshops, as well as a tutorial on related topics, at the above conference venues. She is supported by a Presidential Fellowship at Brown and has interned at DeepMind, Google Research and Microsoft Research.
How Natural Language can Help Multi-Agent Decision Making
Insights from human language, for example, how compositional operators work over reusable concepts, or how humans communicate with one another to solve tasks, provide a rich source of information as to how intelligent behaviour arises. Can this help us build agents that intelligently coordinate with one another to solve complex tasks? In this talk we'll go over three different ways of using language to help multi-agent decision making and game-theoretic analyses of problems. The first focuses on using language as a communicative tool to allow agents to collaborate and solve tasks more effectively. The second shows how we could use supervision in the form of language skills to train agents to coordinate on a task better. The third shows how tying together insights from game theoretic axioms and models of language can allow more intelligent algorithms for language tasks. Lastly, we show how probing agent representations for human-interpretable concepts can help us better understand and explain their behaviours, leading to safer and more interpretable agents.