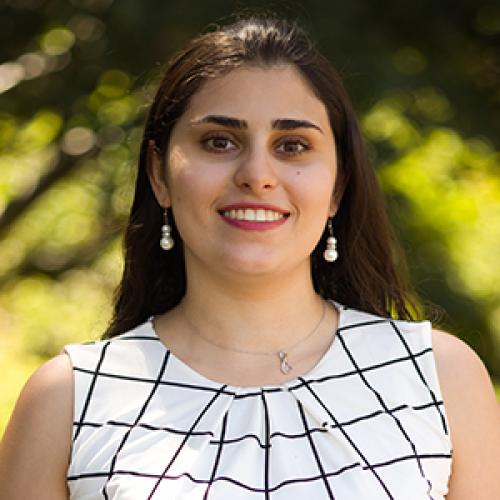
(she/her/hers)
University of California Irvine
Applied machine learning, cyber-physical systems, embedded systems, security, hardware
Rozhin Yasaei is currently a Ph.D. candidate at the University of California Irvine, studying computer engineering under Professor Mohammad Al Faruque. She received her M.Sc. degree in computer engineering from the University of California Irvine in 2021 and her B.Sc. in electrical engineering from Sharif University of technology in 2018. Her research interest lies in data-driven modeling for cyber-physical systems. She applies state-of-the-art machine learning techniques, such as graph neural networks, to hardware and embedded systems for security and design automation. She was selected for the pedagogical fellowship in 2021 and, through one year of training, received a teaching excellence certificate and the qualification to train incoming students as teaching assistants.
As a minority female scholar in the STEM field, Rozhin is devoted to promoting diversity and inclusion and supporting her fellow collages by actively volunteering for service to the community and presenting in various panels and workshops for women in STEM. She has been the president of the UCI chapter of the Women in CyberSecurity (WiCyS) organization since 2021 to promote women's participation in this field. Qualified with mentoring excellence certificate, she has been selected by several programs at UCI to mentor undergraduate and graduate students.
Graph Neural Networks for Cross-Layer Security
The time-to-market pressure and continuous growing complexity of hardware designs have promoted the globalization of the Integrated Circuit (IC) supply chain. However, such globalization also poses various security threats in each phase of the IC supply chain.
Although the advancements in Machine Learning (ML) have pushed the frontier of hardware security, most conventional ML-based methods can only achieve the desired performance by manually finding a robust feature representation for circuits with non-Euclidean data.
As a result, modeling these circuits using graph learning to improve design flows has attracted research attention in the Electronic Design Automation (EDA) field. However, due to the lack of supporting tools, only a few existing works apply graph learning to resolve hardware security issues.
Therefore, we propose the first methodology based on the graph neural network that models the hardware design as a graph and achieves state-of-the-art performance on two major security issues: Hardware Trojan Detection} and Intellectual Property Piracy Detection.
Our proposed method provides an automated pipeline for extracting a graph representation from a hardware design at various abstraction levels (register transfer level or gate-level netlist).
Besides, it automatically transforms the non-Euclidean hardware designs into Euclidean graph embeddings to facilitate solving security issues. The embeddings are further exploited to detect similarities between hardware designs and IP theft or malicious manipulation of designs known as Trojan.
Our approach has introduced a new way of modeling hardware and inspired many works in the domain. Due to our graph learning-based approach's superior performance and promising potential, we further study its application in cross-layer security issues such as Internet of Things (IoT) security.