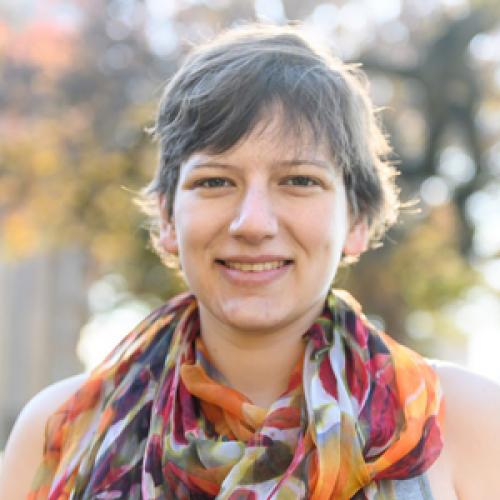
(she/her/hers)
MIT
Human-AI Interaction, Human-Robot Interaction, Explainable AI, Human-Centered Reinforcement Learning
Serena Booth is a PhD student in MIT CSAIL's Interactive Robotics Group. Serena aspires to design better robot and AI collaborators by enabling agents to explain their behavior and by giving people the tools to change agent behavior. Serena is supported by an MIT Jacobs Presidential Fellowship as well as the NSF Graduate Research Fellowship Program. Outside of research, Serena has served as President of MIT's Science Policy Initiative, where she aims to increase the representation of scientists in government and the legislative process. Before joining MIT, Serena was a Product Manager at Google, where she worked on search and augmented reality.
Supporting Conceptual Model Development for Human-Robot and Human-AI Interaction
People should be able to easily understand and change robot and AI behaviors, and experts should be able to easily specify and debug robot and AI behaviors. My thesis explores how we can better support people---both general users and experts---in these tasks. The key insight is that humans need flexible and accurate conceptual models of robot and AI behaviors, but crucially lack them. I will discuss how theories of human concept learning should inform our approach to human-AI and human-robot interaction. I will then discuss specific methods for strategically-selecting examples which communicate these robot and AI behaviors in service of building these conceptual models.