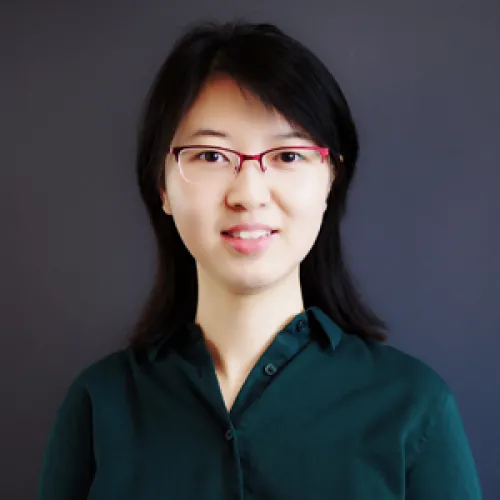
(she/her/hers)
MIT
generative modeling, vision language, embodied AI
I am a final-year Ph.D. student at MIT, advised by Prof. Antonio Torralba. My research focuses on Generative Modeling, Vision Language, and Embodied AI. I am interested in building intelligent agents that can continually learn and interact with the world around them. One aspect of my research is to teach AI systems to exhibit Generalization abilities. My works on AI generalization are highly cited in the field. They have also been reported at news outlets including MIT News. I was awarded the Facebook Research Fellowship, Adobe Research Fellowship, and ICML Outstanding Reviewer.
Learning how to Generalize
Humans readily adapt to new environments and are excellent at inventing feasible approaches for new tasks. To unlock its full potential, AI must exhibit similar generalization abilities. For example, after teaching a robot how to make a cup of coffee, the robot should be able to make a cup of tea without much additional guidance.
My research into AI generalization explores two paradigms: 1) generalization that emerges when training high-capacity models on massive data, and 2) learning explicit modules for composing concepts to enable generalization. These two paradigms are complementary: large data and large models can allow AI to understand many types of complex or rare concepts that are difficult to enumerate explicitly, while explicitly learning generalization can yield more stable and predictable models. AI systems with better generalization are safer for people to use. They have better performance when adapting to new environments and tasks. My research in both directions is important for designing AI's capable of solving a wide range of real-world tasks.