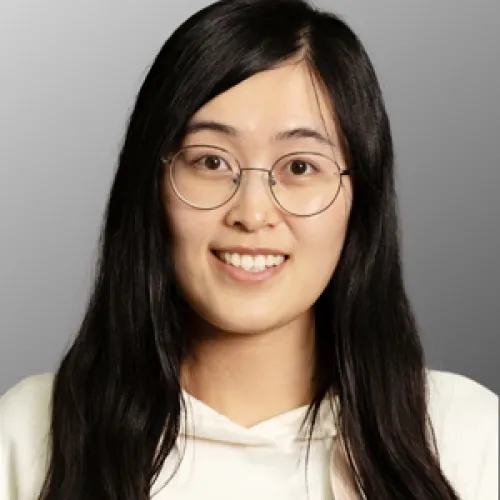
(she/her/hers)
University of Washington
Learning, Control, Optimization, Energy Systems, Theoretical Guarantees
Wenqi Cui is a Ph.D. candidate in the department of Electrical and Computer Engineering at the University of Washington, advised by Prof. Baosen Zhang. Previously, she received the B.Eng. degree and M.S.degree in electrical engineering from Southeast University and Zhejiang University in 2016 and 2019, respectively. Her research focuses on machine learning, control and optimization for cyber-physical power systems. She is a recipient of Rushmer Innovator Fellowship and Clean Energy Institute (CEI) Fellowship at the University of Washington.
Structured Learning and Control for Energy Systems: Stability, Steady-State Optimality and Generalization Guarantees
Increasing the amount of renewable energy sources (RESs) in power systems is of fundamental importance to achieving carbon neutrality. Due to the reduction in rotating mechanical inertia and larger uncertainties, power systems with higher penetration of RESs are more prone to instabilities. My research focuses on control and machine learning methods that apply to large-scale and nonlinear power systems. Most existing learning-based approaches rely on finite samples and soft penalties for hard constraints, which are not convincing enough to system operators. I seek to bridge the gap between neural network-based controller design, stability and performance guarantees, and extend to a wide range of nonlinear networked systems.
I have developed structured learning approaches to optimize both transient and steady-state performances while providing provable stability guarantees. I explicitly engineer the structure of neural network-based controllers such that they guarantee stability for large range of system parameters and topologies. This is done by using a Lyapunov function for power system transient dynamics to guide their structures. I proceed to design structured neural network-based controllers to enforce both transient stability and steady-state optimal resource allocation. Importantly, the proposed approach achieves steady-state optimality distributedly, allowing it adapt to time-varying variables in the system without centralized optimization. To enable true "plug-and-play" integration of RES in large distributed power networks, I further propose a modular approach for network-level stability certification. This allows the performance guarantees of the neural network-based controller generalize to a wide range of networked systems. Together these works provide a systematic framework to achieve both stability and steady-state performance guarantees in learning-based control optimization.