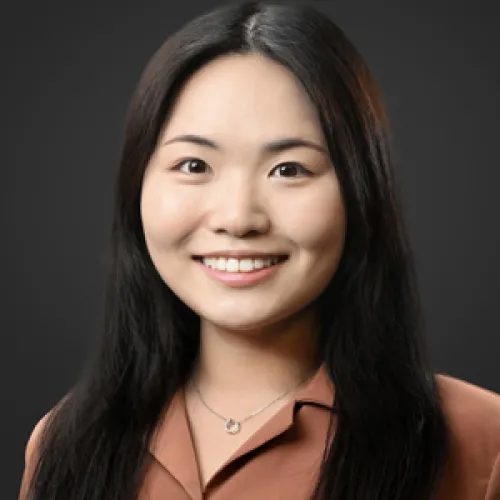
(she/her/hers)
University of California, Berkeley
Machine Learning and Economics, Optimization, Algorithm Design
Wenshuo Guo is a PhD candidate in Computer Science at University of California, Berkeley, advised by Michael I. Jordan. Her research interests lie in the intersection of machine learning, statistics and economics, in particular building machine learning systems that are more robust, equitable, and responsive to practical social and economic needs. Her research has been supported by a Google Ph.D. Fellowship in Algorithms, Optimizations and Markets and a Berkeley Graduate Fellowship. She is affiliated with Berkeley AI Research, the CLIMB Center, and SkyLab. Previously, she received her B.Sc. in Physics, in Mathematics and in Computer Science from Hong Kong University of Science and Technology.
Application-driven incentive-aware algorithm design
Many of the classical machine learning methodologies have focused on optimizing average-case performance in an offline setting, with strong distributional assumptions. However, when learning systems are deployed in the real world, it is important to consider the dynamic social and economic contexts that posit new feasibility constraints, and influence the kinds of data that the systems can actually obtain. I will present some recent work on developing learning algorithms that incorporate these social economic contexts into the design. In the first work, we focus on a new auction model that is motivated by real-world applications: the class of auctions where the information about items is only partially-revealed. We propose an online formulation of these types of mechanisms and present algorithms that are no-regret from the buyer's perspective. In the second part, I will talk about a new framework for policy evaluation and optimization, where the decision-maker's utility is not a simple average of individuals across a population, but is realized as an output of a downstream planning or decision-making problem.