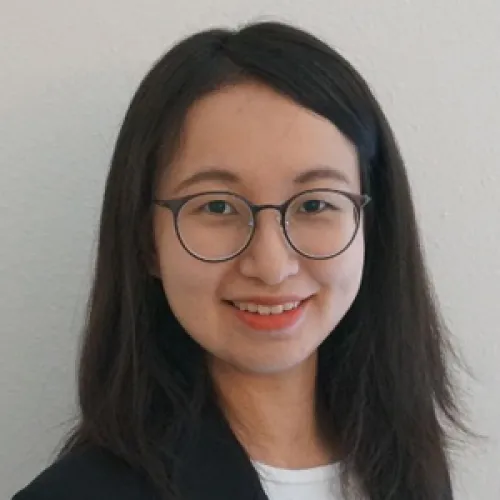
(she/her/hers)
Duke University
In-memory computing, emerging nonvolatile memory technologies, robustness and reliability.
Xiaoxuan Yang is a Ph.D. candidate in Electrical and Computer Engineering at Duke Univerisity, under the supervision of Dr. Hai (Helen) Li and Dr. Yiran Chen. She received the B.S. degree in electrical engineering from Tsinghua University and the M.S. degree in electrical engineering from University of California, Los Angeles (UCLA). Her research interests include emerging nonvolatile memory technologies, robustness and reliability enhancement in processing-in-memory designs, and hardware accelerators for deep learning applications.
Improving the Efficiency and Robustness of In-Memory Computing in Emerging Technologies
In-memory computing can be efficiently implemented using emerging technologies. Resistive random-access memory (ReRAM) is one of the most promising emerging technologies featuring high density, low access energy, and the feasibility of realizing multi-level cell and 3D integration. Prior ReRAM-based Processing-in-memory (PIM) designs have demonstrated their potentials in performing vector-matrix multiplications (VMM) compared with pure CMOS accelerator architectures. However, the previous designs cannot achieve high efficiency in the new and appealing attention-based model. Besides, due to the non-idealities in the hardware, the inferencing accuracy of the in-memory computing system has severe degradation. Therefore, this work aims to improve the efficiency and robustness of in-memory computing with hardware-software (HW-SW) co-design innovations. The contributions of this work are as follows: (1) The architectural design ReTransformer focuses on enabling an efficient self-attention mechanism in the ReRAM-based in-memory computing system. (2) The system-level design with stochastic-noise-aware training algorithm aims to tolerant device stochastic noise and find Pareto-optimal solutions for PIM systems concerning accuracy and hardware efficiency. (3) The algorithm solution HERO targets building robust networks against weight perturbation with Hessian-enhanced robust optimization.