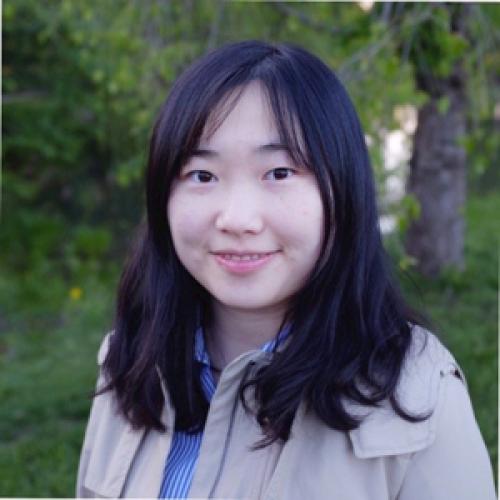
(she/her/hers)
Dana-Farber Cancer Institute and Harvard University
Computational biology, cancer genomics, machine learning, bioinformatics, human genetics
Dr. Yi Zhang is a data science research fellow at Dana-Farber Cancer Institute and Harvard University. She obtained her Ph.D. in Bioengineering from University of Illinois at Urbana-Champaign in 2019 working with Dr. Jun S. Song. During her Ph.D. studies, she led development of computational methods to understand why some human genetic variants are associated with higher cancer risk, using genomics and epigenetic data integration. Yi then joined Dr. Shirley X. Liu's lab to develop machine learning methods to understand single-cells in tumor. She has led computational research published in Cancer Research, Bioinformatics, Frontiers, Blood, and co-authored publications in Cell, Neuro-oncology, and Nature Machine Intelligence. Her current research focus is bioinformatics and computational biology, and specially computational methods development for large-scale multiomic and biomedical data using machine learning. She is devoted to use computational approaches to better understand complex human diseases such as cancer.
Data-driven understanding of the single-cell space in tumor microenvironment
Recent advances in single-cell RNA sequencing revealed common cell types and states in the tumor microenvironment (TME), which are related to cancer progression and therapy response. However, tumor scRNA analysis relies on clustering cells and annotating with markers from experts. Challenges include inconsistent cell types or states definition, different marker selection among studies, batch effect, and that clustering doesn't find continuous cell states. All of the above limitations from current methods create large barriers on consistent and high resolution understanding of tumor compositions.
We developed a data-driven framework, MetaTiME, to overcome the limitations in resolution and consistency. MetaTiME, in short of Meta-components of Tumor immune Microenvironment, is based on machine learning and integrates datasets on the level of subspace rather than on the level of data points. Using millions of TME single cells, MetaTiME learns independent components of gene expression observed across cancer types. The method not only integrated single-cell datasets, but also transfer knowledge across tumor single-cell studies, generating an understandable space of cell states.
We found that the MetaTiME-generated space is highly interpretable, forming meta-components representing cell type, cell states, and signaling pathways in tumor microenvironment. The fully annotated MetaTiME space is useful in three aspects. First, top genes of each MeC direction provide co-expressed gene modules, such as multiple cancer immunotherapy target genes. Thus, the MeCs overall form a landscape of cell states infiltrating tumor, represented by the gene modules. Second, by projecting onto the MetaTiME space, we provide a tool to annotate cell states and signature continuums for TME scRNA-seq data. Third, leveraging epigenetics data, MetaTiME reveals critical transcriptional regulators for the cell states. Overall, MetaTiME learns data-driven meta-components that depict cellular states and gene regulators for tumor immunity and cancer immunotherapy.